Knowledge VQA
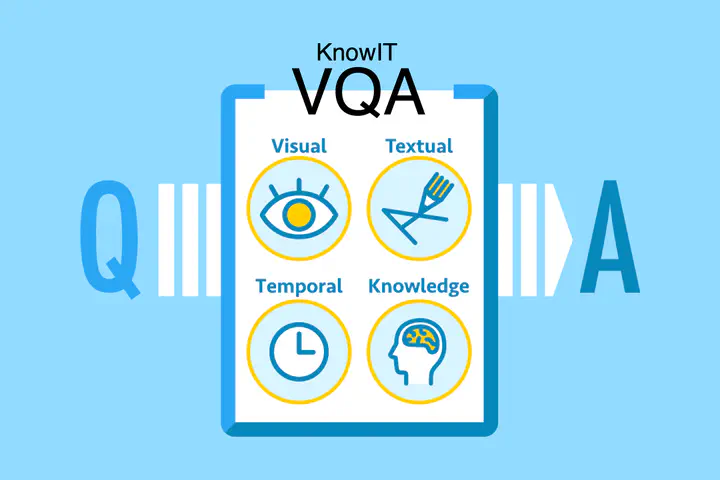
Visual question answering (VQA) with knowledge is a task that requires knowledge to answer questions on images/video. This additional requirement of knowledge poses an interesting challenge on top of the classic VQA tasks. Specifically, a system needs to explore external knowledge sources to answer the questions correctly, as well as understanding the visual content.
We created a dedicated dataset for our knowledge VQA task and made it open to the public so that everyone can enjoy our new task. The results are presented at AAAI 2020.
Representation of videos has been a major research topic for various deep learning applications including visual question answering. This is a challenging problem especially for tasks that involves vision and language and some researchers pointed out that deep neural network-based models mainly use natural language text but not the vision. We propose to use textual representation of videos, in which SOTA models for detection/recognition are used for generating text together with some rules. The results are presented at ECCV 2020.
We also work on question answering on art, which requires high-level understanding of paintings themselves as well as associated knowledge on them.
Publications
- Noa Garcia, Chentao Ye, Zihua Liu, Qingtao Hu, Mayu Otani, Chenhui Chu, Yuta Nakashima, and Teruko Mitamura (2020). A Dataset and Baselines for Visual Question Answering on Art. Proc. European Computer Vision Conference Workshops.
- Noa Garcia and Yuta Nakashima (2020). Knowledge-Based VideoQA with Unsupervised Scene Descriptions. Proc. European Conference on Computer Vision.
- Noa Garcia, Mayu Otani, Chenhui Chu, and Yuta Nakashima (2020). KnowIT VQA: Answering knowledge-based questions about videos. Proc. AAAI Conference on Artificial Intelligence.
- Zekun Yang, Noa Garcia, Chenhui Chu, Mayu Otani, Yuta Nakashima, and Haruo Takemura (2020). BERT representations for video question answering. Proc. IEEE Winter Conference on Applications of Computer Vision.
- Noa Garcia, Chenhui Chu, Mayu Otani, and Yuta Nakashima (2019). Video meets knowledge in visual question answering. MIRU.
- Zekun Yang, Noa Garcia, Chenhui Chu, Mayu Otani, Yuta Nakashima, and Haruo Takemura (2019). Video question answering with BERT. MIRU.